DL Pipeline
DL model implementations obey a clear design principle of flowing specific processes. It is important to mention the sequence of tasks at this point so that the analysis of the technology and the reference to discrete software can take on the importance they deserve.
The workflow in the development of a DL model starts with data collection. Then, this data is cleaned and processed so that later the appropriate labeling or the definition of input variables can be done depending on the problem we are asked to solve. Then comes the stage of transforming the input data. Since this research deals with DL model implementation and ANN, the input data i.e. the set of features from the dataset are transformed into tensors. The input data is represented by tensors and as such it is input to the ANN to perform the model training. After the training is completed, i.e. when the ANN has read all the input data then the evaluation of the model is performed, i.e. a necessary process through which by means of specific measurements we can identify the error rate in its predictions. In essence, to check if the training has gone well or to improve the process further. The final process is storing the trained model and turning it into a productive environment on some decorator in the network.
Lifecycle
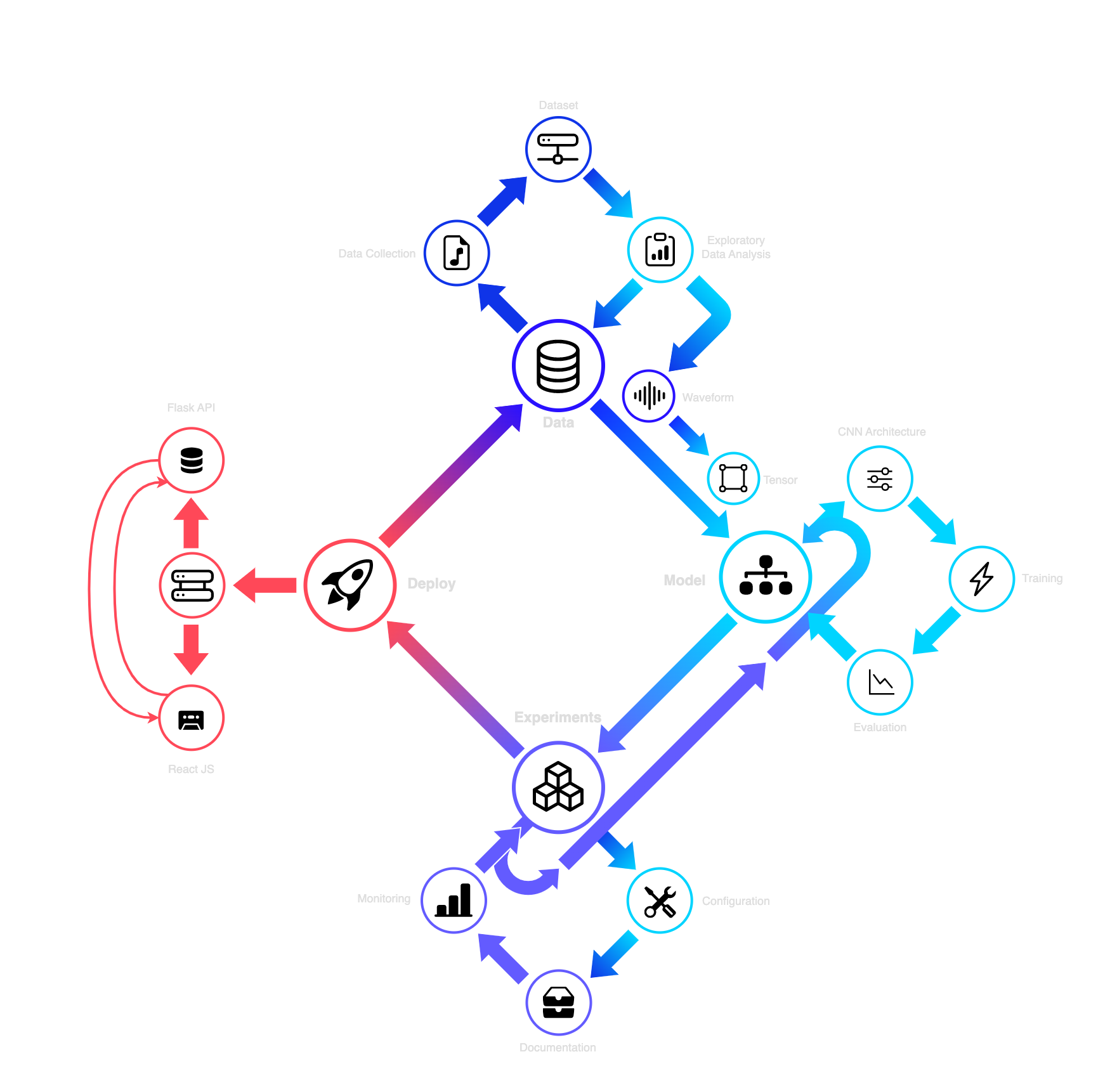