Experiment 1
Binary classification with VGGish model architecture. Logs and metrics have been implemented with tensorboard and pytorch.
Parameters
basic setting parameters
clip_length: 1.0 # [sec]
preprocessing parameters
sample_rate: 22050
hop_length: 512
n_fft: 1024
n_mels: 64
Training parameters
number of audio samples: 22050
learning rate: 0.001
batch size: 10
number of epochs: 10
number of samples: 60
balanced dataset: True
random clip cut: False
Classes
Labels
grime
jazz
Class distribution
Class | Class ID | Samples |
---|---|---|
grime | 0 | 30 |
jazz | 1 | 30 |
Results
Logs
...
Epoch: 10 [30/60 (50%)] Loss: 0.591532 Accuracy: 73.33333333333333%
Epoch: 10 [60/60 (100%)] Loss: 0.585686 Accuracy: 70.0%
[Class: grime] accuracy: 86.7 %
[Class: jazz] accuracy: 56.7 %
Epoch: [10/10] Loss: 0.588609 Accuracy: 71.66666666666667%
[[=============================================================================================]]
Metrics
Cross entropy
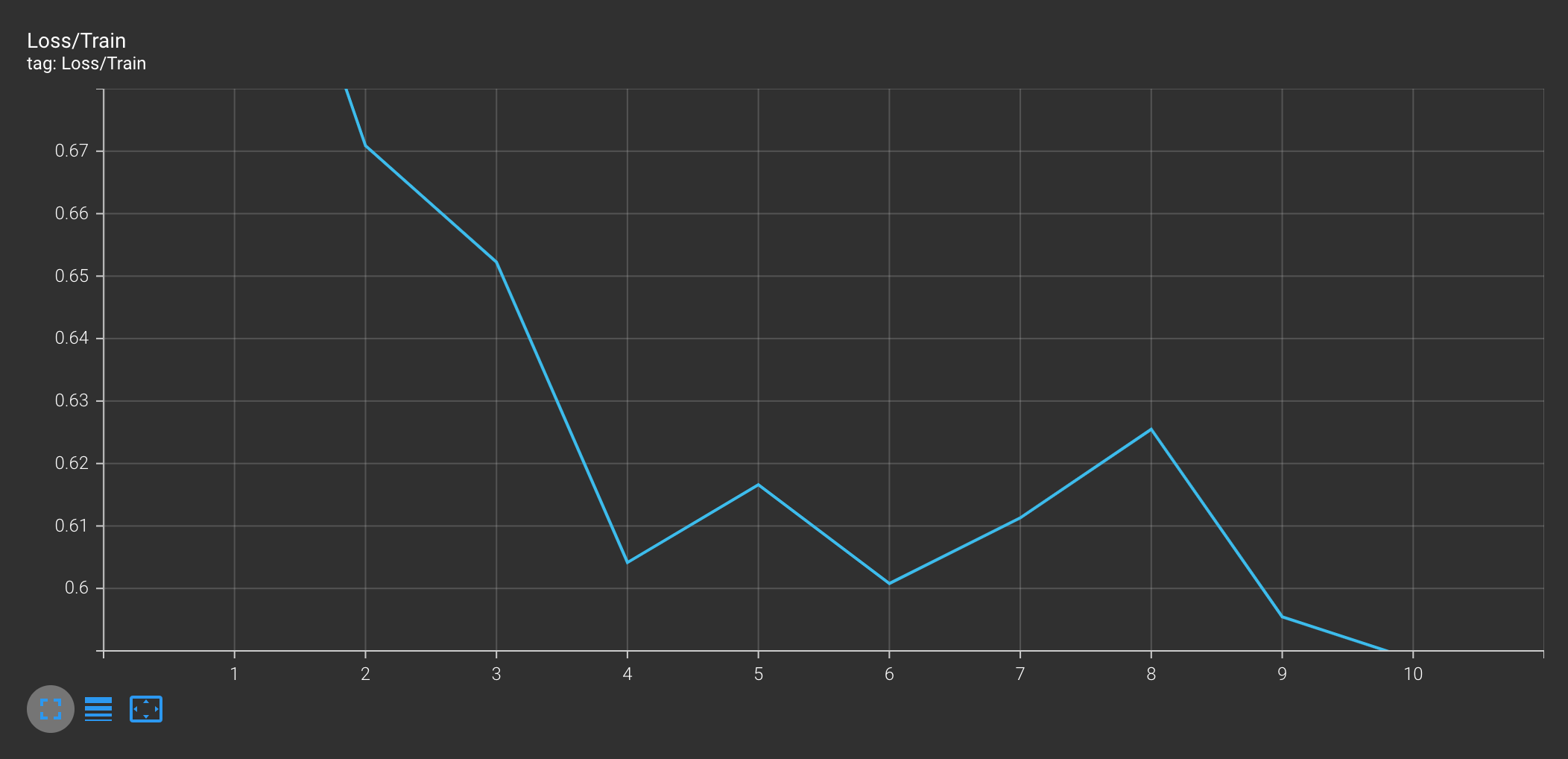
Accuracy
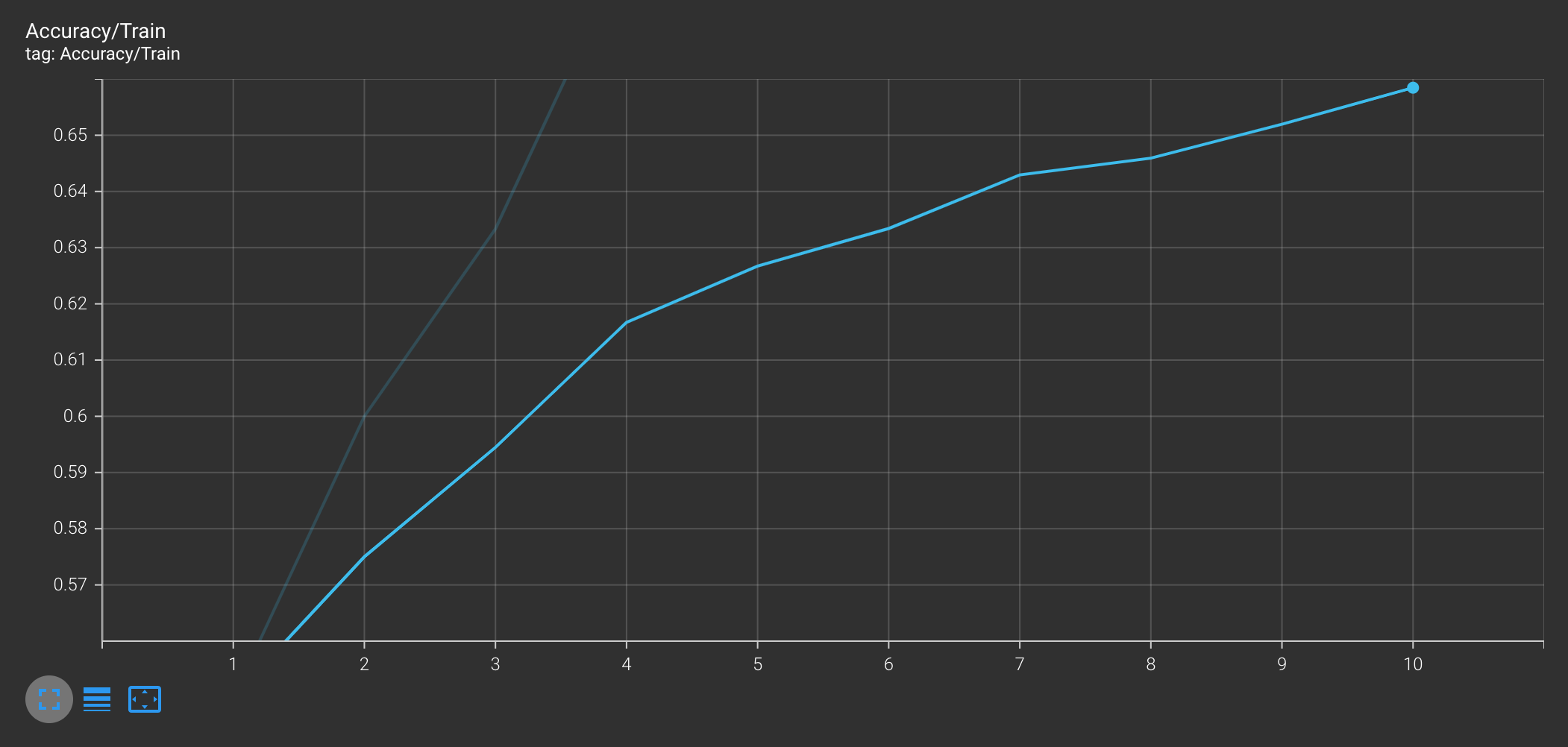
Loss/Accuracy per batch
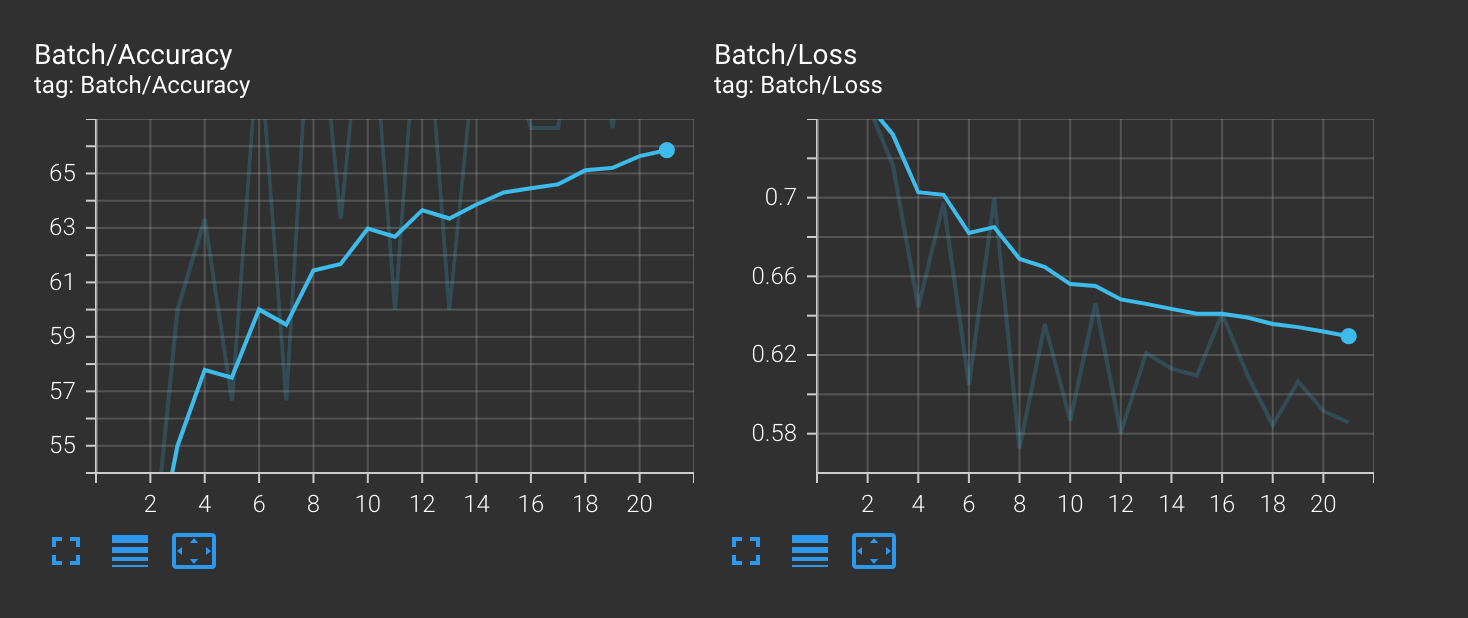
Accuracy per class
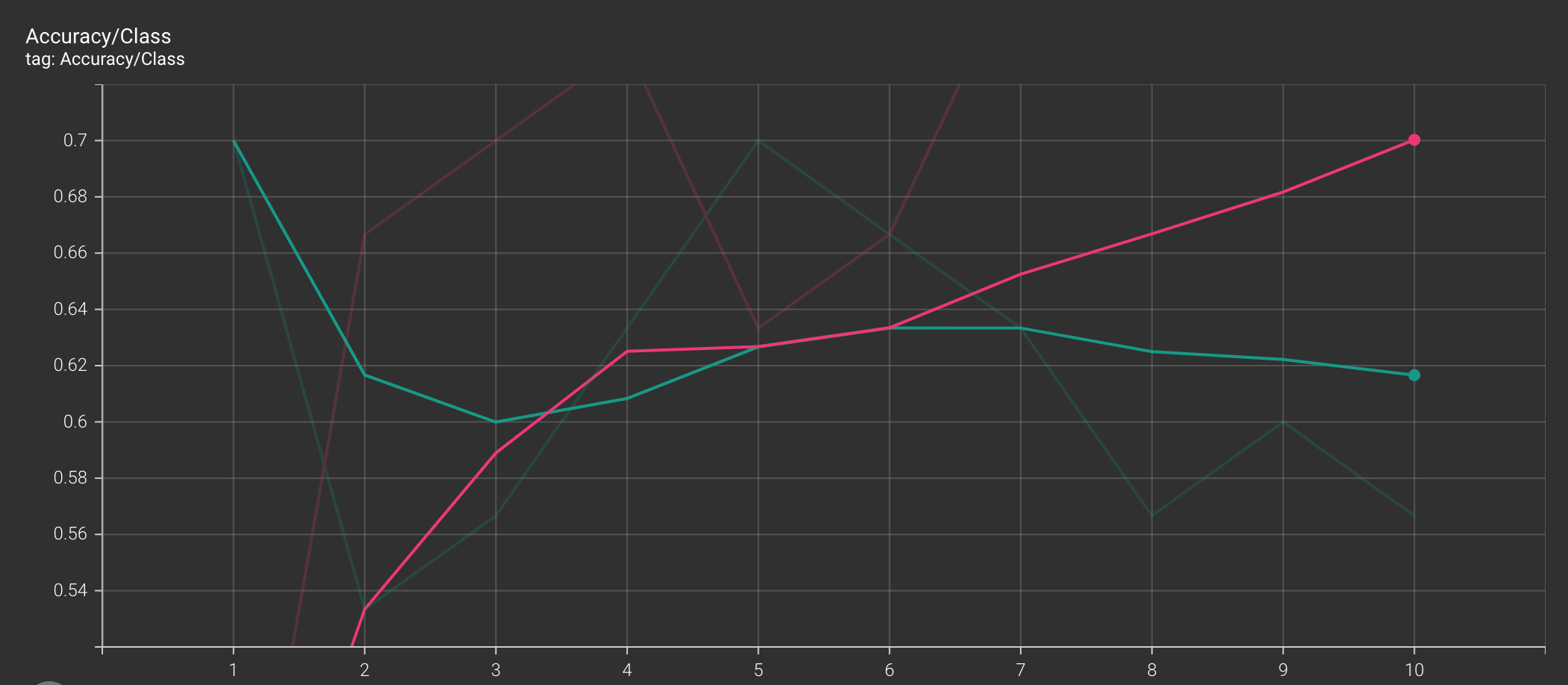
Conv4 Layer
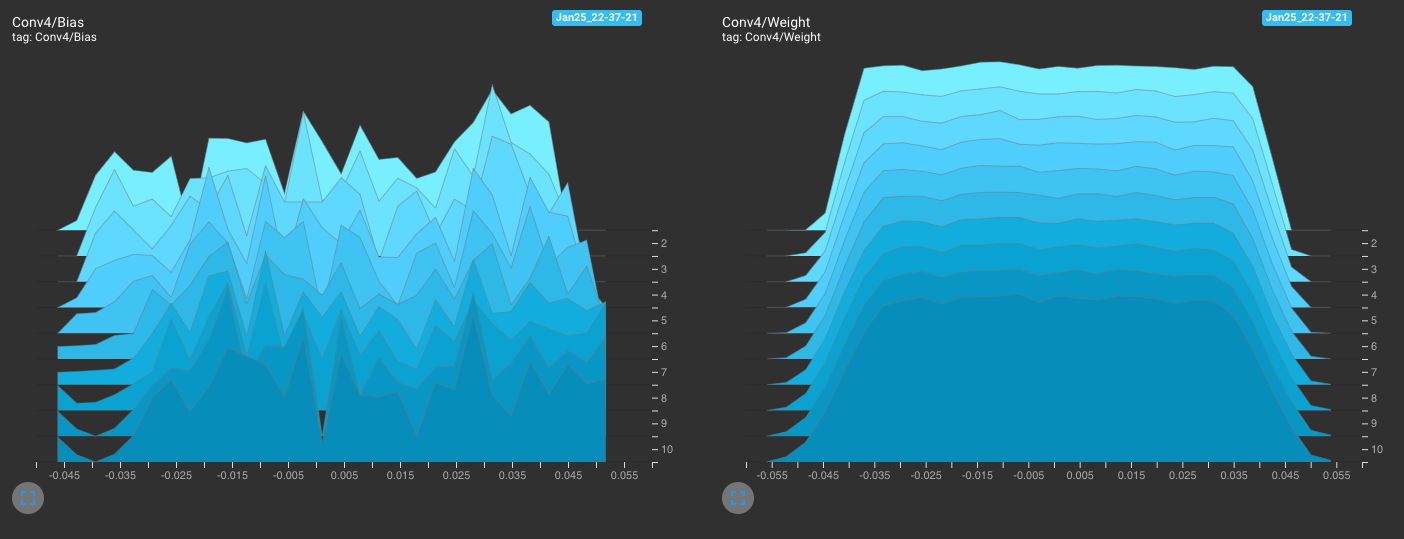
Linear layer
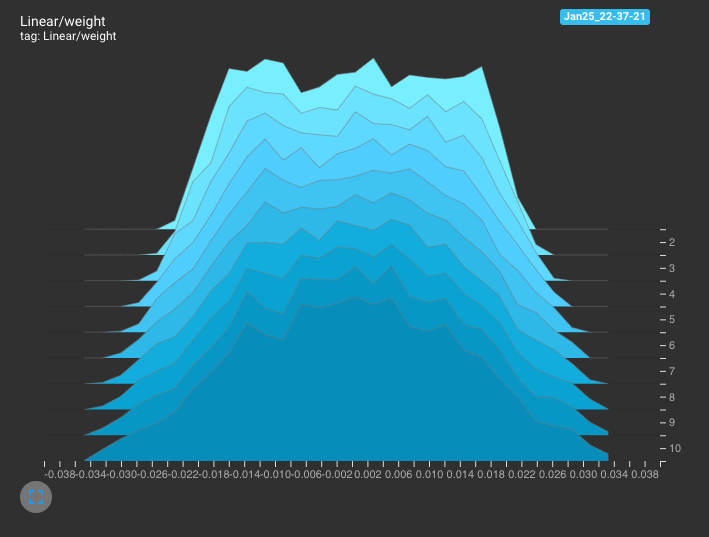
Confusion Matrix
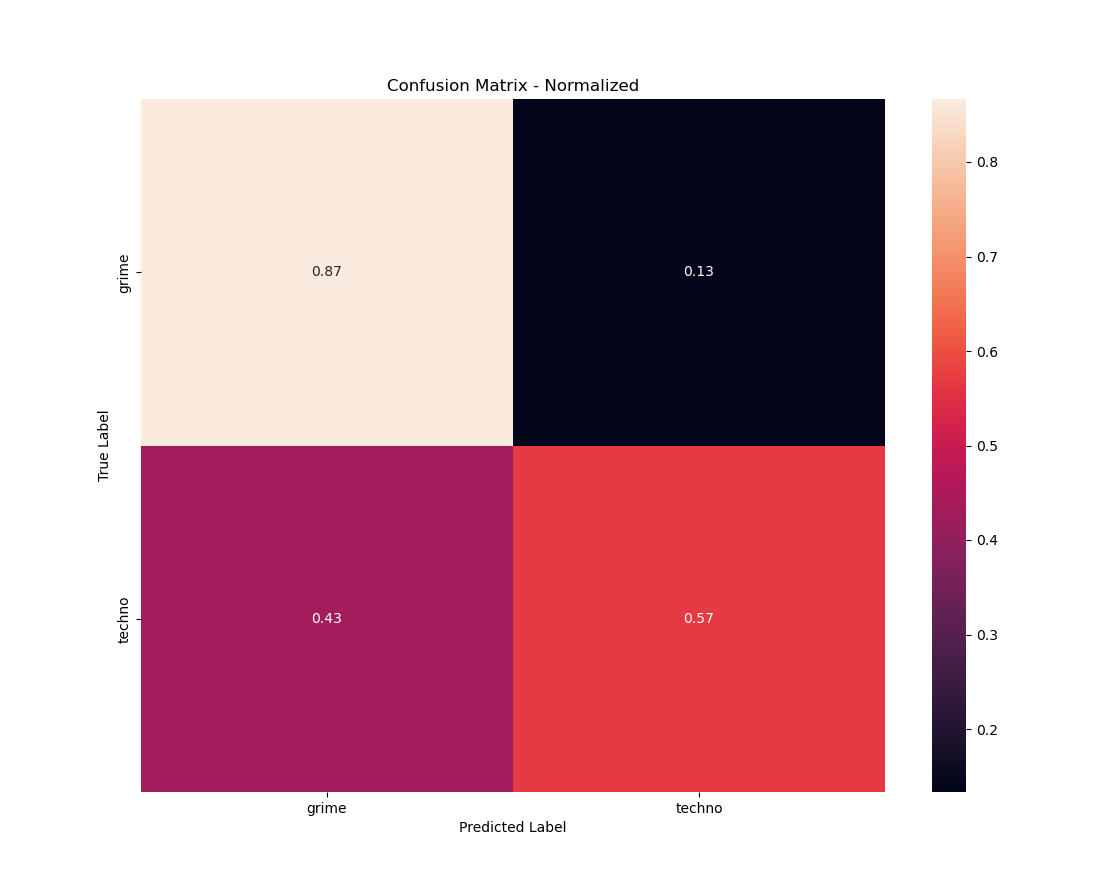